Being Watched: How surveillance amplifies racist policing and threatens the right to protest — Don’t Call Me Resilient EP 10 transcript
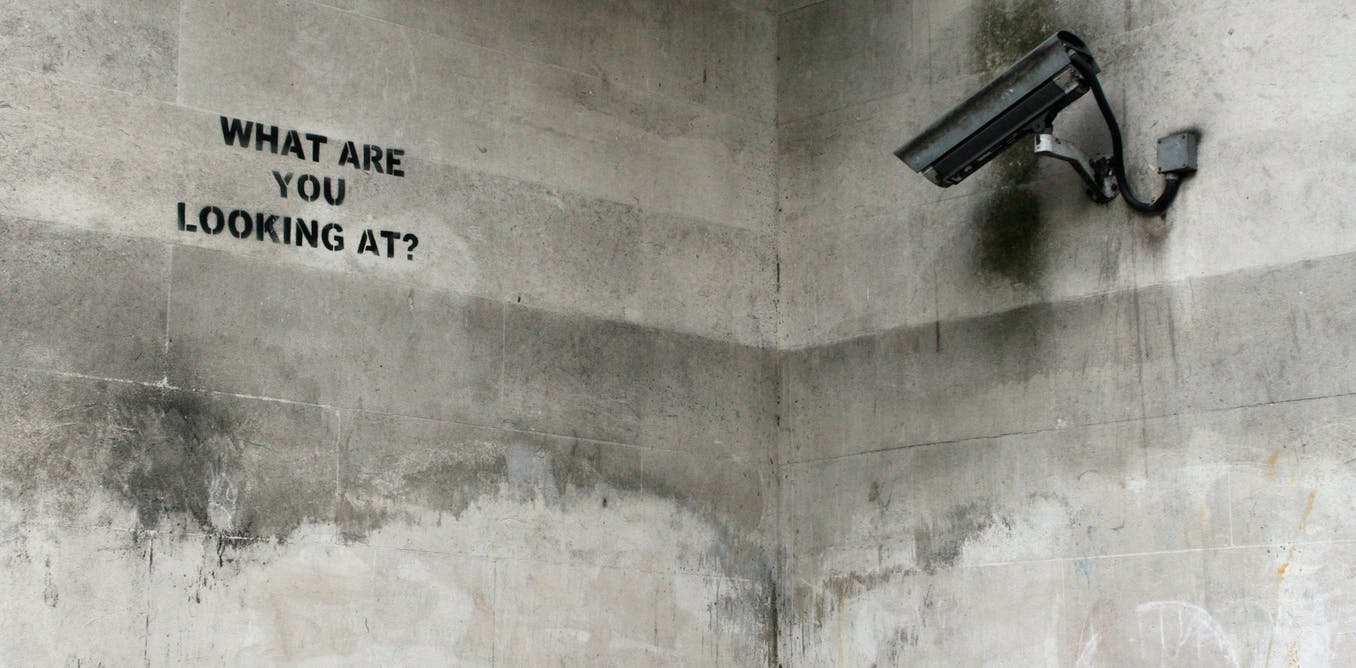
A photo of art work by Banksy in London comments on the power imbalance of surveillance technology. Guests on this episode discuss how AI and Facial recognition have been flagged by civil rights leaders due to its inherent racial bias. Niv Singer/Unsplash
Episode 10: Being Watched: How surveillance amplifies racist policing and threatens the right to protest.
NOTE: Transcripts may contain errors. Please check the corresponding audio before quoting in print.
Vinita Srivastava (VS): From The Conversation, this is Don’t Call Me Resilient, I’m Vinita Srivastava.
Wendy Hui Kyong Chun (WKC): We don’t have to accept the technology that we’re given. We can reinvent it, we could rethink it. We need to challenge the defaults.
VS: It feels like technology, like facial recognition and artificial intelligence, are an inevitable part of our lives. We ask Google Nest or Alexa to find and play a song. We use our faces to unlock our phones and we share news articles on social media. I’ll be honest, I feel like this technology has its upsides, like when it can track and predict climate change or identify the rioters who stormed the U.S. Capitol. But there are also a lot of downsides. Once analysts gain access to our private data, they can use that information to influence and alter our behaviour and choices. And like most else, if you’re marginalized in some way, the consequences are worse. Experts have been warning about the dangers of data collection for a while now, especially for Black, Indigenous and racialized people. And this year, Amnesty International called for the banning of facial recognition technology, calling it a form of mass surveillance that amplifies racist policing and threatens the right to protest. So what can we do to resist this creeping culture of surveillance? Our guests today are experts in discrimination and technology. Yuan Stevens is the Policy Lead on Technology, Cybersecurity and Democracy at the Ryerson Leadership Lab and a research fellow at the Centre for Media, Technology and Democracy at McGill School of Public Policy. Her work examines the impacts of technology on vulnerable populations in Canada, the U.S. and Germany. Wendy Hui Kyong Chun is the Canada 150 Research Chair in New Media at Simon Fraser University and she leads the Digital Democracies Institute there. She’s the author of several books, including Discriminating Data, which is out this fall. I’ve been thinking non-stop about surveillance and facial recognition for the last little while, as you can imagine. I’m not living under a rock. I know that there are significant dangers around personal data collection. And yet I’m one of those complacent people. I’ve got two kids. I’ve got a full-time job. I’m really busy. And I actually love social media. I put pics of my kids on there last night. So what are some of the risks of sharing my life online? Yuan, what do you think?
Yuan Stevens (YS): I think there is a lot at stake when it comes to the amount of data we’re giving companies and how they can treat us and what they can do with that data once they have it. So what I do in my work is I basically look at the development of technology and I think about the ways it can be abused. One of the worst possible outcomes is that we end up in a place where companies work with governments to have this data and to access this data, but to also categorize us and control us. So one of my own personal interests is how people were treated by the Stasi and by their peers in the German Democratic Republic. And I think about how different they think about their data than we do in North America because they have a history behind them of the state snooping into their lives. There’s this ethnographic study by a researcher named Ulrike Neuendorf, and she was able to discover that the impacts of this surveillance included things like significant impacts on their well-being, mistrust and significant trauma. If you think about what it feels like for someone to know something about you that you didn’t want them to know, that is huge.
VS: What I’m hearing you saying is that this has implications for our health, our lives, our well-being and society. I sort of understand it on a large scale, that it can result in all of these things that are troubling. But on a personal level, what are the dangers there for somebody who is like, well, I’m a law-abiding citizen, so what’s the problem?
WKC: So I think that one thing we can do is maybe switch it a little and not say I’m a law-abiding citizen. What’s the problem? But ask, what are the conditions under which you are a law-abiding citizen? So what’s really fascinating now is the example you started with. You took pictures of your kid. You put them online. What’s wrong with this? What’s interesting now is that publicity and surveillance are so intertwined now that it’s hard to understand their difference. So in other words, when you take that picture and you put it up and you create a public persona, you engage with people. It’s not simply you putting it up, but the ways in which by you doing this, what else is happening?
VS: I’m just going to use an example as old, old school in the ‘90s when I was an activist on campus, we knew that they were Canadian agents somewhere in our midst. We just knew that they were collecting files on us. But in my head, I imagine those files to be like manilla folder files with black and white photos. So information was just more localized. And I don’t know what it’s like to be an activist today. And I’m wondering about the — especially for racialize people, queer people, immigrants, refugees — how they might be extra targeted by this kind of information and surveillance. What’s at stake for these communities?
YS: Yeah, I think it’s a really good question of who stands to be, I think, the most targeted and harmed by the use of surveillance technology. So whether you’re queer or a religious minority or person of colour or if you are protected under discrimination law, what that means is that you deserve treatment that ensures that your rights are protected in the same way that you would be if you were a dominant group. It’s absolutely true that certain groups are going to be more targeted than others. So if you look at predictive policing technologies, there are certain logics inscribed in the use of and design of those technologies that can further perpetuate realities or statistical findings that existed before. So, for example, if you decide to deploy police to a certain neighbourhood because there are more instances of crime there, in fact, what you could be doing is finding crime more often there, primarily because you’re actually sending police there more than if you were to send them to another neighbourhood, for example.
VS: You’re just looking more basically.
YS: Exactly. That’s one of the instances in ways in which people of colour, for example, and racialized people can be further subject to surveillance and further found guilty of crimes because what you have is a feedback loop. So feedback loops are a really important concept when you’re looking at surveillance studies in the context of technologies.
VS: Every time I think of predictive policing, I’m thinking about this dystopian movie, Minority Report.
WKC: So a classic example of this is the Chicago heat list, which is now no longer being used. And there, they came up with — allegedly — they said what we’re doing is just coming up with a list of people most likely to be murdered or to murder somebody and then we’re going to go visit them and say, “look, you better change your ways or else something bad is going to happen.”
VS: Oh, my God, that dystopian movie is actually real.
WKC: It is real.
YS: Absolutely.
WKC: And the way that they determined the people most likely to be murdered was by going to past arrest history. So if you had a co-arrest with somebody who became a homicide victim, that would be a strong indicator that you then would be involved in a homicide. Now, what’s really strange about this is that, first of all, they didn’t take time into consideration. So you have these people who had co-arrests from being a kid and when marijuana was illegal, smoking weed together, who had clean records being visited by police and saying, look, you have to change your ways. And since some of these people had clean records when the police came and visited, the neighbours were like, this guy’s a snitch. The crazy thing as well is that the data that went into these predictive policing models and the whole setup of the model itself came from studying mainly African-American neighbourhoods in the west side of Chicago. So race and background are already there. So race didn’t need to be an overt factor because it was an implicit factor. So if you think of how these programs work, they’re trained using certain data in the way that they’re validated as correct — to say, OK, yes, it’s made a proper prediction — is by hiding some of that past data and then saying, “OK, let’s use this model.” Does it predict the past correctly? So these don’t actually predict the future. They’re tested on the ability to break with the past. So if the past is racist, these programs will only be considered to be correctly validated as accurate if they make racist predictions. So you’re caught in a system in which learning means repeating the past, which means you lose the future. So the reason why we don’t want these automated systems is because all it does is automate past mistakes. So some artists did this great mock-up of a machine-learning programme to find the white collar criminal on the fancy side of New York, blah, blah, blah. So, I mean, I think that the question is, how are we understanding exactly what Yuan was talking about, which are the communities that are most policed so we have the most data about? And so, if the police really want to say, look, we want to be effective and we want to use our resources, then go for this empty swath of people in the suburban homes, doing all sorts of stuff that are never pulled over or looked into. Not that I’m advocating for that.
Click here to listen to Don’t Call Me Resilient
VS: I want to talk a little bit about Clearview, because some of this became known when the story of Clearview broke in the mainstream media that all of our data is scraped and then put into this database that is now being used for facial recognition and this database being sold to police or to law enforcement or to companies. Can you explain a little bit about that case and why it’s so important in Canada, the Clearview case?
YS: Yeah, absolutely. What happened was this company, a start-up that’s still getting funding, tried to provide and is trying to provide its services to the general public and to the police and to governments and all kinds of entities. Clearview AI is a facial recognition technology company, but it’s also a data scraping company. So what it does is it scrapes data from all kinds of sources, social media websites in general, collects those, has used deep learning and machine learning technologies to analyse whose face is whose and categorise those. And then what it does is it sells the service of matching faces. Why this matters is not only is the company selling essentially face matching capabilities but it’s scraped significant amounts of data contrary to law that would otherwise prevent the scraping of data. Now scraping it in itself as not to be seen as criminal. I think it can be used for legitimate reasons, for example, by academic researchers. But none of this is done without our consent. We had no notice. We had no knowledge of this.
VS: You mean like Canadians. When you say we, we’re talking about residents of Canada?
YS: Yeah, I think when it comes to both racism and surveillance, we do have Canadian exceptionalism and Clearview AI and its use by the RCMP is another example to show that surveiling and the surveillance of us in Canada absolutely exists and is occurring. The reason why it matters too is because what happened was the RCMP was using Clearview AI services and conducted hundreds of searches, though it only admitted to some of those to the office of the privacy commissioner. And it’s always about the child predators. It always starts with that. And that’s something that Bruce Schneier has referred to as the four horsemen of the info apocalypse. Which is this idea that there is certain aberrant behaviour that you want to address. And then you say, you know, I’m going to use this technology only in those situations, which could be true. All of us can get behind the idea that children should be protected. And that’s, of course, I believe that, too. But then what you see happening is surveillance creep and the ability to use that same technology in other situations. And that’s actually, in a way, what’s happening potentially or what could happen with Apple scanning our images before they’re stored in our iCloud for, again, child sexual abuse materials. People who are concerned about how technology can be used and abused are always thinking in a sort of Minority Report sense. And the good reason we’re trying to see what is the absolute worst that can happen with us is because we’re trying to protect all people, because you know that in order to protect all people, you can’t allow certain people to be treated a certain way necessarily unless they’re … depending on how much trust there is an institution.
VS: Are you basically saying my photos in my phone are also something to be worried about?
YS: Absolutely, absolutely.
VS: Just gets worse and worse. We have to talk for a minute now, or more than a minute, about facial recognition. I know that you both look at this in your work. Can we talk a little bit about what the technology is and also how it’s being used right now, Wendy?
WKC: Sure. So facial recognition technology is a form of pattern recognition. And so it’s the idea that somehow and these are done through machine learning programs mainly, and they don’t focus on features that make sense to us. It’s not like a computer saying, “oh, I remember these people’s eyes, I’m going to match this eye to that eye,” but rather through various algorithms. Basically, you see one face and you try to match it to another face is the basic technology. It’s very problematic. It doesn’t really work well. It’s also very bad because the early programs were trained on publicly available faces. So you’re thinking Hollywood. Now think of what a hotbed of diversity Hollywood is. Other ones are like undergraduates who will do anything for five dollars or some school credit. So the libraries were mainly white. And so these technologies work very well with light-skinned faces and really poorly with dark-skinned faces. It’s getting better. But that’s not the point. The point isn’t that this needs to be perfect for all skin tones. But the reason why this matters so much is that think of how self-driving cars operate. If they can’t recognize dark people as people, then there’s clear danger that’s involved in this. But also because it’s not refined on dark-skinned faces. And this is something that people at Georgetown (University) have been working on a lot, is that it will misrecognize dark faces as criminal because it doesn’t have that distinction. So there was this famous example given by the ACLU where they looked at the U.S. Congress and said who amongst these are criminals? And it was disproportionately people of colour that were marked as “criminals.”
VS: So basically, these technologies are built on historical information, which includes historical discrimination, historical racism. And so, this idea that science is neutral and technology is neutral is completely wrong. Basically, the discrimination is built into the technology.
YS: Yeah, to the point, work by Kate Robertson and Cynthia Khoo at Citizen Lab has shown that we absolutely do have a bias to believe that mathematical processes are neutral. And so we’ll trust technology and we’ll want to listen to it, so to speak, when it has a certain output. And it’s because we think that this is statistics, this is maths. I don’t understand how it works, it must be fine. And that’s really problematic when you consider the fact that not only police but judges could also rely on essentially recommendation systems. It’s probably OK that we can be recommended some TV shows and Netflix before certain recommendations to be made regarding the most fundamental of our rights, that is a totally different story.
VS: So should we just completely be not using this technology at all?
YS: I absolutely think there should be certain no-go zones when it comes to the collection and particularly the processing of our data for certain outcomes. So, for example, in the general data protection regulation, which is one of the most advanced and progressive data protection regulations in Europe, what is not allowed is the processing of information for automated decision-making for the purposes of profiling. On its face, what that suggests to me is that you shouldn’t be allowed to, for example, collect information about faces in the public setting, perhaps are very certain circumstances. But the presumption should be that you don’t collect faces and biometric information in the setting and therefore to render someone potentially criminal and biometric information is also a really sensitive data type that I think that absolutely deserves special protection. Right now in Canada, there isn’t special affordances given to the protection of that kind of data. What we have is this kind of free for all in a way where all data is the same. But in fact, different kinds of data have different levels of sensitivity and there should be enforceable regulation in Canada saying that spelling out the kinds of data that should not be treated in certain ways. And right now that doesn’t exist.
VS: And Wendy, what were you going to say?
WKC: I completely agree with everything that Yuan has said. I want to just talk about the predictive part of this, because what I would argue is that the problem is using these programs for prediction. The famous example is Amazon’s hiring algorithm, which was trained on all of the hires it made. And what ended up happening is if you had “woman” anywhere on your CV, you lost.
VS: How is that even possible? The technology actually docks you a point for being a woman?
WKC: Yeah. So because they went by who they hired and who they didn’t hire. They didn’t hire women, so clearly being a woman is bad, you’re not going to be a good employee. And so they ditched the program. But rather than ditching it, what if we said thank you so much for meticulously documenting your discrimination? We use these not for prediction, but actually as evidence of historical trends. The example I always give is global climate change models. So global climate change models give you the most probable future given past and present behaviour. But then we don’t say, “oh, this is great, it’s going to go up two degrees, let’s make it go faster,” or we’re offered the most probable future so we won’t make that future happen. So what if we took a lot of these things which are allegedly predictive and said, OK, the heat list shows Chicago police are discriminatory, so let’s make sure that the kinds of things that would be automated under this don’t happen. So I think that’s one thing. Take these and look at them as historical probes rather than as predictive. To offer one example of people who are doing this, at USC in the Geena Davis Institute, they’re using these kind of pattern recognition technologies to go through the past archive of Hollywood films and to see what kind of gender representation is there and think through what kinds of representation there have been within mainstream media.
YS: Yeah, and maybe in a more hopeful note as well, I’m aware of efforts by the Algorithmic Justice League, which looks at how people can flag issues with algorithms with respect to how they’re biased. And the hope is that you can improve systems because you say this is something that should be fixed and there are risks inherent with opening up your systems for criticism by the public. But I think it’s really one important step forward to allowing people who are affected by these technologies to impact their design that would actually give rise potentially to what Sasha Costanza-Chock calls design justice. And I won’t go into that in-depth here, but it’s really the idea that there’s meaningful participation of community groups in the design of technology.
VS: So just talking about the participation of groups in the creation of technology, I don’t know what it’s like for a protester right now on the street. But I do know that summer of 2020 we had uprisings in the United States, but also in Hong Kong and Beirut. And I know that facial recognition is not just used in North America. It’s a global issue that we’re talking about this idea of surveillance. And I know that both of you have talked about some of the ways that the protesters have resisted the surveillance. What caught your attention, Wendy, with some of these protests?
WKC: Well, what’s important is that they’re very aware of how the technology works, because, again, what we started with is the ways in which publicity and surveillance are now intertwined. So it’s hard to think through publicity without thinking through surveillance at the same time. And what I would argue that the protesters show us and that we need to start thinking about our public rights, because I really think the work that is being done around privacy is important, but it’s completely inadequate. And there’s a thought that once you’re in public, you lose all rights, you’re simply exposed. You’re a public figure. But increasingly we’re all public figures. And what we need to be able to do is to be in public, vulnerable, and yet not attacked. And what I find really important is the ways in which people offer each other shade, either through making sure pictures are taken in a certain way or people registering that they’re at a certain place in order to provide a larger or different sense of location for these technologies. And these are inadequate in terms of long term solutions. But what they bring out is if you think again of how all these recommendation engines work or how everything works, we’re fundamentally intertwined with each other. Everything you get is based on what somebody else has done, which means we’re fundamentally connected. So what if we took this position of being connected as a place from which to act and to act collectively and to say we need to be able to loiter in public because everybody should have the right to loiter, everyone should have the right to be in public. And if we switch it this way, I think that this opens up an entirely different conversation. And more importantly, it moves privacy away from corporations get to know everything about you, but not share to other users and think about it in a far more expansive way.
VS: You said provide shade, is that what you said? Provide shade for others, for each other?
WKC: Yes, literally and metaphorically. And this comes from a lot of the work that Kara Keeling has done. She’s in African-American studies and in film studies. And we have been trying to think together through this question of exposure, shade and protection. And it comes from work that she’s done in analysing slavery and in house enslaved women took care of each other and their bodies, not because they own them, but because they did it, because they were outside of certain notions of privacy. So privacy, especially within the U.S., is very white. It’s like the first case in New York State around privacy was the protection of Abigail Robinson, I believe, who is a white woman whose photograph was used to sell flour against her will. But while this case was going on, Nancy Green, who was Aunt Jemima, had no rights to her image. She was completely viewed in public. And so I think if we move away from certain notions of privacy, which have never been adequate, and instead think through publicity as an enabling position that isn’t based on notions of certain really problematic notions of property. I think this can open things up in really productive ways.
VS: I like that the right to loiter, the right to be public, the right to be in the public.
WKC: And that comes from work done by wonderful Indian feminists who wrote a book, Why Loiter, which is all about that feminist women need and Muslim men need the right to loiter in public.
VS: I never really thought about it in that way, the idea of loitering being a right to take up space. But you’re saying we all should have it.
YS: I absolutely agree with Wendy that we have a system in Canada that is actually very similar to the U.S. where we prioritize privacy. In fact, it isn’t just privacy that is at stake, but it’s the right to control our information. The German Constitutional Court calls this informational self-determination. And that phrase to me really encompasses and cuts across a lot of these issues we’re talking about today because we’re talking about privacy, we’re talking about algorithmic decision-making recommendation systems. But privacy alone isn’t enough to protect our rights. Right now, we have changes to Canada’s privacy laws, and that doesn’t go far enough. And in fact, what we need is a comprehensive approach that protects our right to informational self-determination and views us not as consumers, but views us as humans whose human rights are at the core of the most important thing to protect. And that matters because if you’re out in public and if the police are using what’s called an IMSI catcher. So something that can ping to cell towers to tell where you are. Yes, it’s your privacy at stake. But yes, it’s also your freedom to protest. At the root of it is the right to have your information treated in the way that you want to be treated.
VS: Before we wrap up, do you have a couple of top things that you want to leave listeners with, either things that you think individuals should be paying attention to or things that we should look at from a policy level or just observations that you think we should be making?
WKC: We don’t have to accept the technology that we’re given. We can reinvent it. We could rethink it. We need to challenge the defaults. And secondly, technology isn’t the solution to our social problems. It’s often framed this way because there’s this belief that somehow we humans are inadequate and we can build this thing that can take care of these problems for us. It will never be, but it can be, part of the solution. But only if we look at the technology closely and we realize that the technology itself is built-in with these assumptions. But it’s also built on studying certain populations. And that maybe one way, therefore, to change these technologies is to revisit the populations that were so key to the building of certain presumptions, like go back to that residential study of segregation in the United States, realized there was something more and so much more that was happening. And so, therefore, start with everybody we touch whenever we use these technologies as a way to open up different worlds.
YS: And to add to that, I really want to encourage any listeners who care about these topics to take up space too. And this extends what you were saying, Wendy, about the right to loiter and the right in some ways to take up that space. I really would encourage people who would deploy technology, whether you’re policymakers or are the police, to really consider what is in the public interest. And part of the consideration of what’s in the public interest is consideration of how your technology will impact those equality-seeking groups. So it’s twofold. It’s really take up more space if you are going to be a person who’s impacted by this. And also keep in mind the public interest and those are qualities seeking groups when you are using this technology to the detriment of those people.
VS: Lovely to speak with you both so much. Thank you very much for taking the time today to be with me.
WKC: Thank you for inviting us. And it’s a wonderful conversation.
YS: Thank you so much. I’m really honoured to be part of this.
VS: That’s it for this episode of Don’t Call Me Resilient. Thanks for listening. I’d love to know, are you as freaked out as I am after that conversation? Talk to me. I’m on Twitter @WriteVinita. And don’t forget to tag our producers @conversationca. Just use the hashtag #DontCallMeResilient. If you’d like to read more about the creeping dangers of surveillance, go to theconversation.com/ca. It’s also where you’ll find our show notes with links to stories and research connected to our conversation with Yuan Stevens and Wendy Hui Kyong Chun. Finally, if you like what you heard today, please help spread the love. Tell a friend about us or leave us a review on whatever podcast app you’re listening to us on. Don’t Call me Resilient is a production of The Conversation Canada. It was made possible by a grant for journalism innovation from the Social Sciences and Humanities Research Council of Canada. The series is produced and hosted by me, Vinita Srivastava. Our producer is Susana Ferreira. Our associate producer is Ibrahim Daair. Reza Dahya is our incredibly patient sound producer and our fabulous consulting producer is Jennifer Moroz. Lisa Varano leads audience development for The Conversation Canada and Scott White is our CEO. And if you’re wondering who wrote and performed the music we use on the pod, that’s the amazing Zaki Ibrahim. The track is called Something in the Water. Thanks for listening, everyone, and hope you join us again. Until then, I’m Vinita. And please, don’t call me resilient.