Beyond today’s crowdsourced science to tomorrow’s citizen science cyborgs
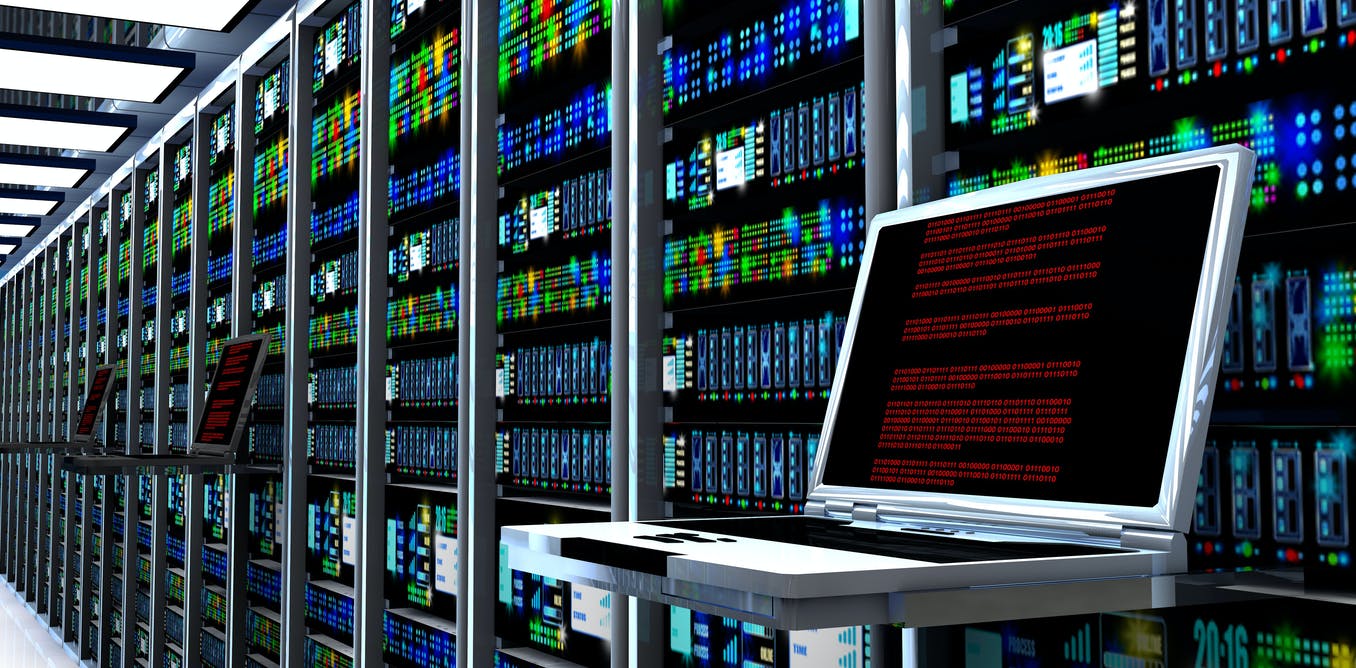
All that computer power will still need a helping hand from our uniquely human expertise. Computers image via www.shutterstock.com
Millions of citizen scientists have been flocking to projects that pool their time and brainpower to tackle big scientific problems, from astronomy to zoology. Projects such as those hosted by the Zooniverse get people across the globe to donate some part of their cognitive surplus, pool it with others’ and apply it to scientific research.
But the way in which citizen scientists contribute to the scientific enterprise may be about to change radically: rather than trawling through mountains of data by themselves, they will teach computers how to analyze data. They will teach these intelligent machines how to act like a crowd of human beings.
We’re on the verge of a huge change – not just in how we do citizen science, but how we do science itself.
The awesome human brain
The human mind is pretty amazing. A young child can tell one human face from another without any trouble, yet it took computer scientists and engineers over a decade to build software that could do the same. And that’s not human beings’ only advantage: we are far more flexible than computers. Give a person some example images of galaxies instead of human faces, and she’ll soon outperform any computer running a neural net in classifying galaxies.
I hit on that reality when I was trying to classify about 50,000 galaxy images for my Ph.D. research in 2007. I took a brief overview of what computers could do and decided that none of the state-of-the-art solutions available was really good enough for what I wanted. So I went ahead and sorted nearly 50,000 galaxies “by eye.” This endeavor led to the Galaxy Zoo citizen science project, in which we invited the public to help astronomers classify a million galaxies by shape and discover the “weird things” out there that nobody knew are out there, such as Hanny’s Voorwerp, the giant glowing cloud of gas next to a massive galaxy.
Enter the deep minds
Computer scientists have made significant steps forward in machine learning over the last few years, and some of their inventions have started to hit the public consciousness.
“Deep neural networks” (or deep minds) learn in a way that is closer to how our brains learn. They try to model data – say, photos of people – by turning them into high-level abstractions using multiple layers where each different layer may focus on different tasks (hence the “deep” in deep neural nets).
These machines are learning in a way that is more akin to what humans do: they start to develop their own intuition. Games are where computer scientists put their machine lab rats to the test. With their learned artificial intelligence, machines are starting to intuit which moves in a game are better than others. This is fundamentally different from previous approaches where the computers would try to “brute force” the game by calculating as many moves as possible and use smart statistics to figure out the best move that way.
Watch a deep mind totally ace ‘Space Invaders’
After just four hours of game play, a deep neural net developed by Google’s DeepMind has managed to come up with a Space Invaders strategy so optimal that it was better than any person’s strategy.
Recently, the team behind Google’s DeepMind has thrown down the gauntlet to the world’s best Go players, claiming that their deep mind can beat them. Go has remained an intractable challenge to computers, with good human players still routinely beating the most powerful computers – until now. Just this March AlphaGo, Google’s Go-playing deep mind, beat Go champion Lee Sedol 4-1.
Do we still need citizen science?
We’re now entering an era in which machines are starting to become competitive with humans in terms of analyzing images, a task previously reserved for human citizen scientists clicking away at galaxies, climate records or snapshots from the Serengeti. This landscape is completely different from when I was a graduate student just a decade ago – then, the machines just weren’t quite up to scratch in many cases. Now they’re starting to outperform people in more and more tasks.
Rather than replacing citizen scientists, though, machines can help them – and it could not have come at a better time. Scientific experiments are flooding researchers with data: astronomers needed the help of the Internet to classify one million galaxies from an astronomical survey that took place in the 1990s and 2000s. Soon telescopes like the Large Synoptic Sky Telescope will give us images of billions of galaxies in addition to supernovae, asteroids and other strange things that go bump in the night.
How will astronomers be able to deal with all these data, many of which are time-sensitive? After all, if something goes “bump” and fades quickly, we’d want to try to study it more before it disappears forever. That’s where the machines can really help us: deep minds can scale up to process large data sets if we just give them sufficient processing power and memory.
Citizen science cyborgs
But the machines still need help – our help! One of the biggest problems for deep neural nets is that they require large training sets, examples of data (say, images of galaxies) which have already been carefully and accurately classified. This is one way in which the citizen scientists will be able to contribute: train the machines by providing high-quality training sets so the machines can then go off and deal with the rest of the data.
There’s another way citizen scientists will be able to pitch in: by helping us identify the weird things out there we don’t know about yet, the proverbial Rumsfeldian “unknown unknowns.” Machines can struggle with noticing unusual or unexpected things, whereas humans excel at it.
Hanny’s Voorwerp appears as a blue blob next to a galaxy in this screenshot of the Galaxy Zoo interface. Human citizen scientist Hanny van Arkel noticed it right away, while machines and algorithms trawling through the SDSS survey data did not.
Galaxy Zoo/SDSS/K. Schawinski
Having the citizen scientists help the machines spot these unexpected things in the data would complement the machines’ ability to churn through huge data sets. If a machine got confused by something, or just wanted some extra feedback, it could kick the object back to a human for help, and then update itself to deal with similar things in the future. This could find applications not just in astrophysics, but in many other fields of science, from surveys of the sea floor to archives in museums, and the detectors of particle accelerators.
So envision a future where a smart system for analyzing large data sets diverts some small percentage of the data to human citizen scientists to help train the machines. The machines then go through the data, occasionally spinning off some more objects to the humans to improve machine performance as time goes on. If the machines then encounter something odd or unexpected, they pass it on to the citizen scientists for evaluation.
Thus, humans and machines will form a true collaboration: citizen science cyborgs.
Kevin Schawinski receives funding from the Swiss National Science Foundation. He is the co-founder of the Galaxy Zoo.